In the ever-evolving landscape of finance, predictive analytics stands as a linchpin, reshaping the way to assess creditworthiness. Predictive analytics credit scoring involves leveraging data-driven insights to forecast an individual's credit behavior. This transformative approach is not just a technological marvel; it's a game-changer for the lending industry.
Predictive analytics in credit scoring involves utilizing data-driven insights to forecast an individual's credit behavior. It's a sophisticated tool that employs statistical algorithms, machine learning, and pattern recognition to analyze various data points, such as credit history, income, and other relevant factors. This process aims to predict the likelihood of an individual repaying a loan, offering a nuanced assessment of creditworthiness.
The significance of predictive analytics in credit scoring lies in its ability to enhance the accuracy of risk assessment. By delving deep into historical data and recognizing intricate patterns, predictive analytics empowers lenders to make more informed decisions. This, in turn, allows for tailored financial offerings, improved risk management, and a more precise evaluation of creditworthiness.
As the lending landscape evolves, the influence of predictive analytics becomes increasingly crucial. It provides adaptive solutions that evolve with changing borrower dynamics, aiding in fraud detection, identifying new opportunities, and ensuring regulatory compliance. This blog will guide you through the exciting world of predictive analytics in credit scoring and shed light on how Nected is at the forefront of reshaping this landscape. Let's delve into the future of credit scoring together!
The Need Of Predictive Analytics Credit Scoring
Why is this so crucial? In the dynamic landscape of credit scoring, predictive analytics plays a vital role in shaping the lending industry. Predictive analytics in credit scoring is indispensable, serving critical purposes. Let us take a look on
- Precise Risk Assessment: One of the primary needs fulfilled by predictive analytics is the accurate assessment of an individual's likelihood of defaulting on a loan or credit card payment. By scrutinizing a consumer's credit history, income level, and other pertinent data points, predictive analytics provides a nuanced evaluation of the borrower's creditworthiness. This precision is crucial for lenders in making informed decisions about extending credit while effectively managing associated risks.
- Opportunity Identification: Predictive analytics goes beyond traditional scoring methods, uncovering opportunities to extend credit to individuals who might be overlooked. This inclusive approach ensures that deserving candidates gain access to financial resources, contributing to a more comprehensive and equitable credit landscape.
- Fraud Prevention: In an era where financial fraud is a persistent threat, predictive analytics acts as a powerful deterrent. By analyzing patterns and anomalies in data, it serves as a vigilant guardian, alerting lenders to potential fraudulent behavior. This not only safeguards financial institutions but also enhances the overall security of transactions.
- Regulatory Compliance: Operating in a regulated environment, financial institutions must adhere to stringent compliance standards. Predictive analytics plays a pivotal role in ensuring that lending practices align with regulatory requirements. It provides a proactive means of addressing compliance challenges, mitigating risks associated with non-compliance.
- Continuous Improvement: Beyond immediate assessments, predictive analytics offers a unique advantage by identifying areas for improvement in an individual's credit profile. This forward-looking aspect allows borrowers to make informed financial decisions that positively impact their credit score over time.
In essence, predictive analytics is the cornerstone of well-informed decision-making in the lending sector. It fulfills fundamental needs, including precise risk assessment, opportunity identification, fraud prevention, and regulatory adherence. This understanding empowers lenders to tailor financial offerings with precision, benefitting both institutions and individuals seeking credit.
How Predictive Analytics works in Credit Scoring?
To demystify predictive analytics in credit scoring, let's explore its key components and methodologies. This is not about waving a magic wand but understanding the nuts and bolts that make it tick.

- Data Gathering: Initiate the process by gathering extensive data, encompassing both conventional financial metrics and unconventional sources like social media activities and spending patterns. This diverse dataset forms the foundation for a thorough credit assessment.
- Data Analysis: Following data collection, conduct a meticulous reporting analysis. This phase yields insights into historical trends, patterns, and potential correlations within the gathered information. Lenders gain a comprehensive view of an individual's financial behavior.
- Monitoring: Ensure the ongoing relevance of the predictive model through continuous data monitoring. This step involves vigilant observation of changing trends and adjustments to the model in response to evolving borrower dynamics.
- Predictive Analytics: Advance to the core of the process, which involves predictive analytics. Utilize advanced statistical models and machine learning algorithms to process the data, identifying intricate patterns and relationships for predicting future credit behavior. This approach surpasses traditional scoring models by recognizing subtle signals and correlations.
- Credit Score Prediction: As a result of predictive analytics, lenders obtain a credit score that serves as a numerical representation of an individual's creditworthiness. This score is derived from the analysis of various factors, providing a nuanced and accurate assessment.
This cyclical process of data collection, reporting analysis, monitoring, and predictive analytics empowers lenders to make informed decisions about extending credit. By understanding these intricacies, we demystify predictive analytics in the context of credit scoring, emphasizing its role in fostering more precise and data-driven lending practices. When the financial database is large enough, then normalization of datasets should be included in the process so as to improve the quality of the credit score evaluation.
Methodologies in Predictive Analytics for Credit Scoring
Now, as we saw how predictive analytics in credit scoring works, let us delve into the methodologies that form the backbone of predictive analytics in credit scoring. From statistical approaches like regression analysis and linear discriminant analysis to advanced techniques such as machine learning and behavioral analysis, each plays a crucial role in shaping creditworthiness assessments.

- Regression Analysis: Regression analysis is a statistical method that examines the relationship between one or more independent variables and a dependent variable. In credit scoring, it helps identify the factors significantly impacting creditworthiness. Specifically, logistic regression is often employed, focusing on predicting the probability of default. This technique provides valuable insights into the likelihood of borrowers meeting their financial obligations, a critical aspect of credit assessment.
- Linear Discriminant Analysis (LDA): Linear Discriminant Analysis is another statistical method utilized in predictive analytics for credit scoring. LDA aims to find the linear combinations of variables that best separate two or more classes. In credit scoring, it helps distinguish between creditworthy and non-creditworthy individuals by identifying key variables that contribute to this differentiation. LDA enhances the understanding of the underlying patterns in the data and aids in making informed credit decisions.
- Machine Learning: Decision trees provide a visual representation of decision-making processes, while random forests aggregate multiple decision trees for enhanced accuracy. Gradient boosting is a machine learning technique that builds predictive models by combining the strengths of multiple weak models.
- Pattern Recognition: Pattern recognition is fundamental in predictive analytics, involving the identification of regularities within extensive datasets. This capability allows the system to recognize subtle signals and correlations, uncovering non-linear relationships and hidden trends in the data that may not be apparent through traditional scoring models. It complements traditional statistical methods, providing a more comprehensive understanding of borrower behavior.
- Behavioral Analysis: Behavioral analysis focuses on understanding how individuals are likely to behave based on their historical patterns. In credit scoring, it dissects borrower behavior to gauge how responsibly they are likely to handle financial responsibilities. This includes analyzing spending habits, repayment patterns, and other financial behaviors that contribute to the overall creditworthiness assessment.
By comprehending these key components and methodologies, we unlock the essence of predictive analytics in credit scoring. As we delve deeper into the nuances of this transformative approach, the intricacies of credit scoring become clearer, offering a glimpse into the future of lending assessments. Let's dive into the challenges that predictive analytics has and witness its transformative impact on credit scoring models. Challenges and Solutions in Implementing Predictive AnalyticsPredictive analytics is a game-changer in the world of credit scoring, but like any revolutionary technology, its implementation comes with its own set of challenges. Understanding these hurdles is the first step towards overcoming them. Here, we delve into the common challenges in deploying predictive credit scoring models and how Nected rises to the occasion.
- Data Quality and Quantity:
- Challenge: The success of predictive analytics hinges on the availability and quality of data. Incomplete or inaccurate data can compromise the model's accuracy.
- Solution: Nected emphasizes comprehensive data collection, pulling from diverse sources to ensure a robust dataset. The platform also incorporates data cleansing and validation tools, addressing issues related to data quality.
- Model Complexity:
- Challenge: Developing and deploying complex predictive models can be daunting, requiring specialized expertise.
- Solution: Nected simplifies the process with its user-friendly interface and low-code approach. It empowers users, regardless of their technical background, to effortlessly design, implement, and manage predictive credit scoring models.
- Interpretability and Explainability:
- Challenge: Black-box models can be hard to interpret, posing challenges in explaining decisions to stakeholders.
- Solution: Nected places a premium on model explainability. Users can understand the factors influencing credit scores, fostering transparency and trust. This aligns with the growing importance of explainable AI in regulatory and ethical considerations.
- Overfitting and Generalization:
- Challenge: Models might become too tailored to training data, leading to poor generalization on new, unseen data.
- Solution: Nected employs robust validation techniques and offers features for users to evaluate model performance comprehensively. This aids in generalizing well to ensure reliability in real-world scenarios.
- Deployment Timelines:
- Challenge: Lengthy deployment timelines can hinder the agility of predictive analytics adoption.
- Solution: Nected accelerates the deployment process with its streamlined workflows. Users can seamlessly transition from rules model development to implementation, reducing time-to-value and facilitating swift integration into lending processes.
- Integration with Existing Systems:
- Challenge: Integrating predictive analytics into existing infrastructure can be intricate, posing compatibility challenges.
Solution: Nected offers seamless integration capabilities, allowing users to embed predictive credit scoring models into their existing systems effortlessly. This ensures a smooth transition without disrupting established workflows.
Navigating these challenges is where Nected truly shines. Its holistic approach, from data integration to model deployment and beyond, positions it as a comprehensive solution for predictive analytics in credit scoring. By addressing these challenges head-on, Nected empowers organizations to unlock the full potential of predictive analytics, ushering in a new era of precision and efficiency in credit assessments.
How to implement Predictive Analytics in Credit Scoring
Consider a lending institution aiming to enhance its credit scoring accuracy using predictive analytics and machine learning. Here's where Nected, with its intuitive rules engine and robust features, steps in.
The lending institution, let's call it XYZ Bank, is keen on incorporating predictive analytics to refine its credit scoring models. Nected, with its seamless integration capabilities, takes the lead to help for this transformation.
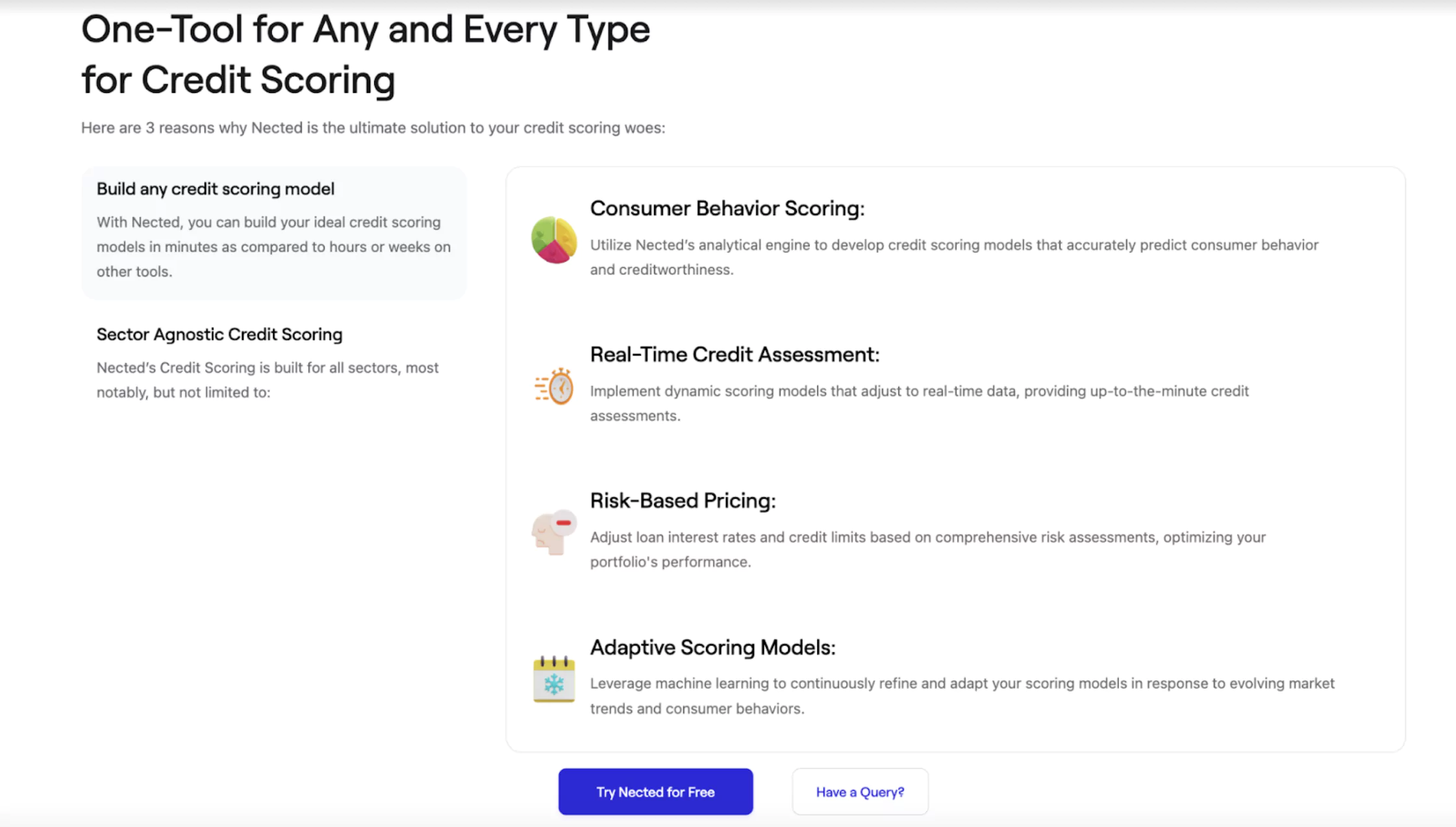
As XYZ Bank adopts Nected, the integration process is simplified. The Amazon Redshift connector allows Nected to pull relevant data directly from XYZ Bank's data warehouse, providing a real-time, comprehensive view of borrower information.
Here is a step by step implementation with Nected:
Step 1: Logging In
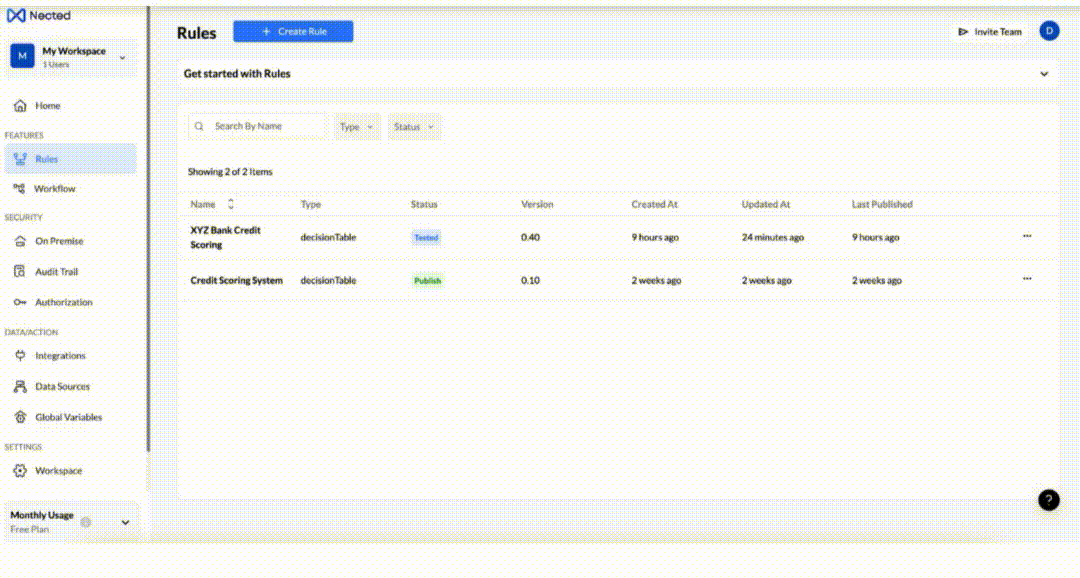
Begin by logging into the Nected platform with your credentials. Access the user-friendly interface designed for intuitive navigation.
Step 2: Versatile Data Integration
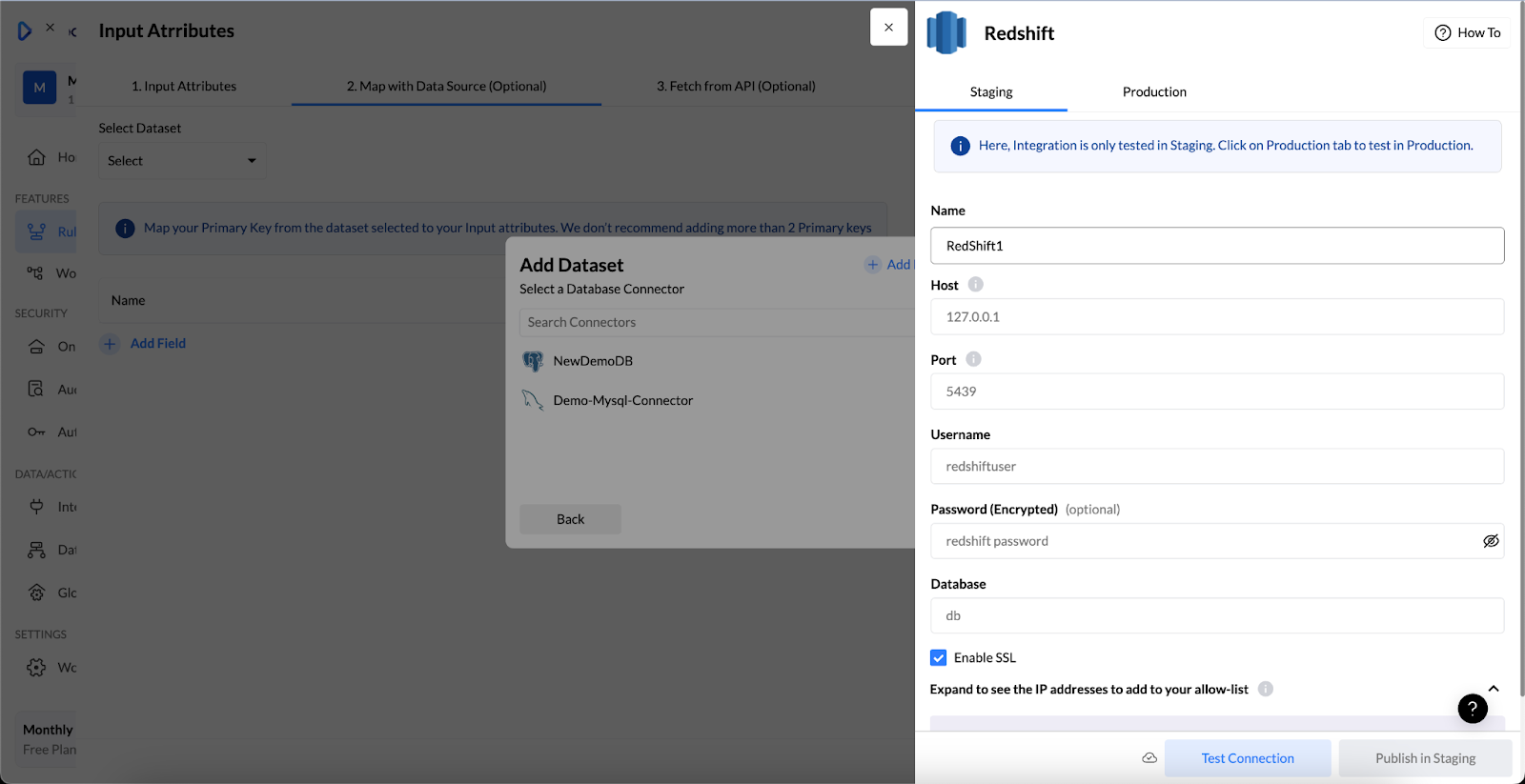
Nected's data integration capabilities shine as you connect seamlessly with Amazon Redshift. Nected's platform versatility is shown by pulling in diverse data from XYZ Bank's data warehouse. From traditional financial data like credit history and income to non-traditional sources such as social media activity, Nected ensures a comprehensive dataset for enhanced predictive analytics.
Step 3: Rule Creation
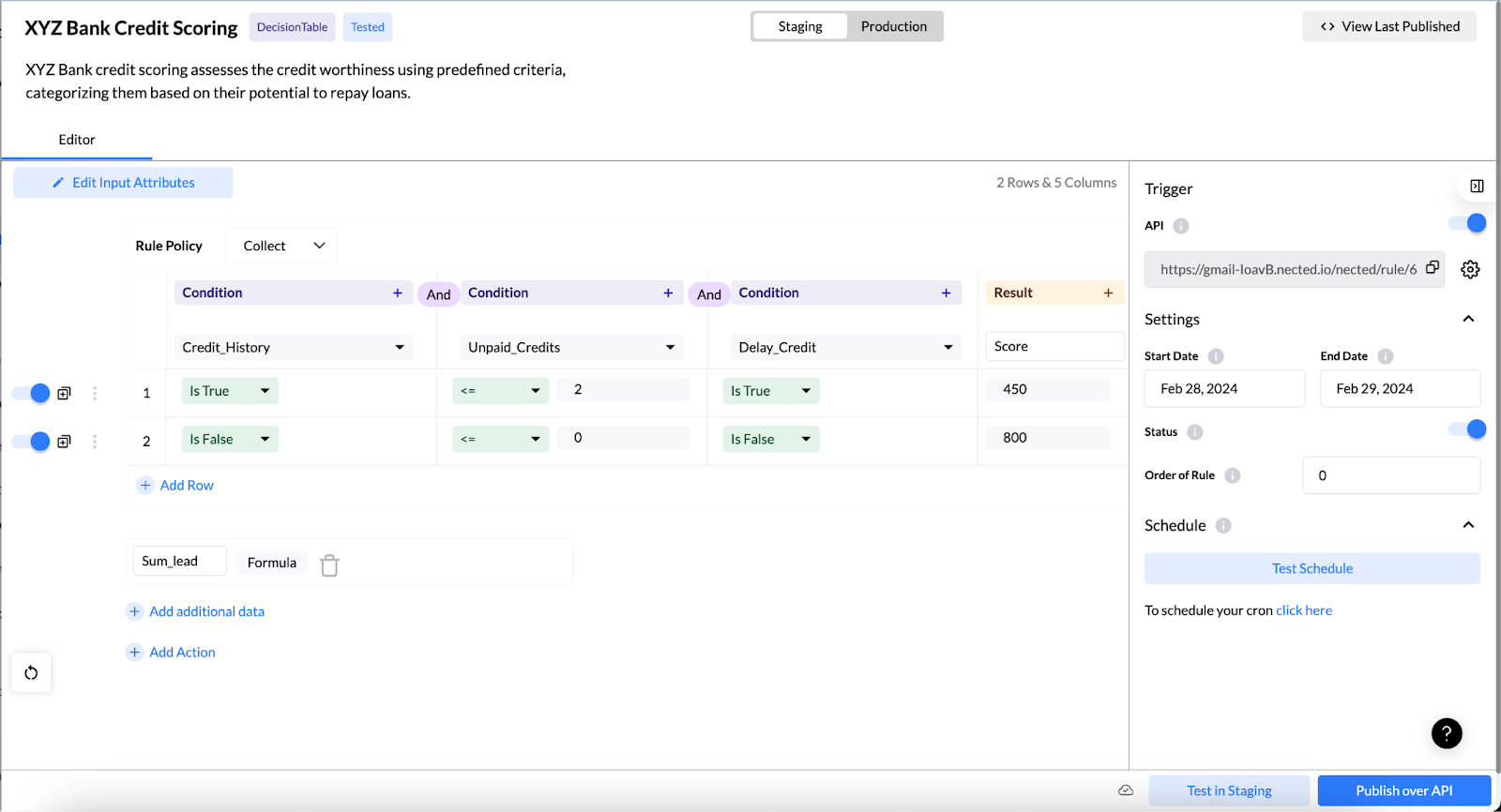
Create a custom rule within Nected to tailor the predictive analytics credit scoring model. Utilize the platform's low-code, no-code features to establish specific parameters aligning with XYZ Bank's credit scoring criteria. This step allows for rule-based customization without the need for extensive coding.
Step 4: Testing and Validation

Rigorously test the developed model within Nected to confirm accuracy and reliability. Ensure that the model aligns with historical data, where outcomes are known, before proceeding to deployment.
Step 5: Deployment

Once satisfied with the testing results, deploy the predictive analytics credit scoring model using Nected. Benefit from streamlined workflows for a swift integration into XYZ Bank's lending processes.

Nected's user-friendly interface facilitates the implementation of predictive analytics in credit scoring without the need for extensive coding or data science expertise. XYZ Bank's team, even without a deep background in machine learning, can configure and use predictive analytics credit scoring models within Nected.
After successful integration of predictive analytics, you have to only make rules based on your business needs which is as easy as a drag and drop game. Just provide rules, test it and deploy it to your application.
In this collaborative scenario, Nected serves as a bridge between predictive analytics, machine learning, and the rich data resources in Amazon Redshift. The result is not just an improved credit scoring system but a transformation in how XYZ Bank approaches lending decisions, embracing data-driven insights for a more inclusive and informed credit environment.
Conclusion
In the dynamic world of credit assessment, predictive analytics emerges as a backbone, propelling lending into a future defined by data-driven precision. In conclusion, exploring predictive analytics for credit scoring reveals a transformative landscape influencing lending decisions. Through data-driven insights, financial institutions gain nuanced decision-making power. This journey demystifies key components, algorithms, and methodologies.
Predictive analytics is more than numbers; it strategically fuses foresight and borrower behavior insights. Beyond technical aspects, it catalyzes improved risk assessment, opportunities, fraud detection, and regulatory compliance. This benefits institutions and contributes to a robust financial ecosystem.
In essence, predictive analytics isn't just a marvel; it's a fundamental shift in lending decisions. Shaped by predictive analytics, the future promises a more inclusive, informed, and visionary credit landscape.
Nected's role goes beyond being a tool - it's a visionary approach to credit scoring that offers operational excellence and adaptive, future-ready models. This exploration concludes with an invitation to financial organizations and decision-makers: embrace the possibilities with Nected. Explore a future where credit decisions are empowered by data, and where Nected is the ally in shaping a credit landscape that's inclusive, informed, and visionary.
Step into a tomorrow where credit scoring is not just a necessity but an opportunity to revolutionize lending decisions. With Nected, the future of credit assessment is not distant—it's within reach.
FAQs
Q1. What sets predictive analytics credit scoring apart from traditional methods?
Predictive analytics credit scoring uses data-driven insights to forecast borrower behavior, offering a more nuanced and accurate assessment compared to traditional approaches.
Q2. Can predictive analytics be seamlessly integrated into existing credit assessment processes?
Yes, Nected provides an intuitive platform, making the integration of predictive analytics into credit scoring both effective and efficient.
Q3. What benefits does machine learning bring to predictive credit scoring?
Machine learning enriches predictive models, offering adaptive solutions, improved accuracy, and the ability to evolve with changing borrower dynamics.